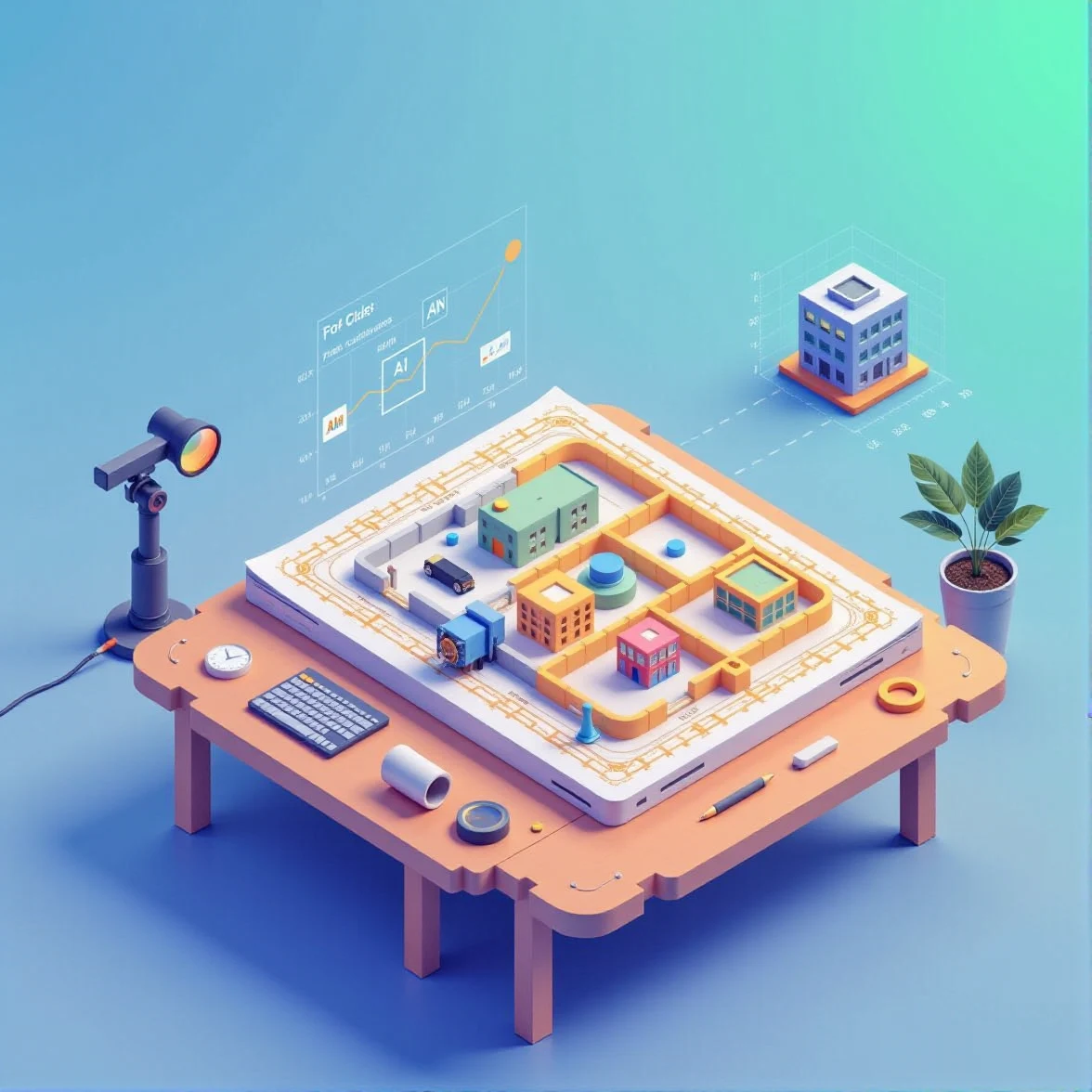
How AI Could Help Processing Point Clouds to 2D Drafts
The architectural, engineering, and construction (AEC) industries are experiencing a rapid transformation as artificial intelligence (AI) becomes an integral part of workflows. One area where AI is proving particularly useful is in the conversion of point clouds into 2D drafts. Point clouds, generated through laser scanning or photogrammetry, consist of millions of data points representing the geometry of a space or object. While rich in detail, these datasets are complex and require significant time and expertise to process manually.
By integrating AI into this workflow, professionals can significantly accelerate the processing of point cloud data, improve accuracy, and reduce the costs associated with manual drafting. In this article, we’ll explore how AI technologies can enhance the process of converting point clouds into 2D drafts and what this means for the future of the AEC industry.
The Challenges of Manual Point Cloud Processing
Converting point cloud data into usable 2D drafts is a labor-intensive process that requires specialized software, skilled drafters, and significant time investments. Some of the common challenges include:
1. Data Complexity
Point clouds are vast datasets containing millions or even billions of points, making them difficult to interpret without advanced tools and expertise.
2. Noise and Irrelevant Data
Raw point clouds often include noise, such as points from objects outside the area of interest. Cleaning this data manually is time-consuming.
3. Accuracy Demands
Creating precise 2D drafts requires careful tracing and alignment of point cloud data with project specifications. Errors can result in costly rework or miscommunication.
How AI Transforms Point Cloud to 2D Drafting
Artificial intelligence brings a host of capabilities to streamline and enhance the process of converting point clouds into 2D drafts. Here’s how AI is making a difference:
1. Automated Data Cleaning
AI algorithms can automatically identify and remove irrelevant points, such as noise or outliers, from raw point cloud data. This reduces the time and effort required for manual cleaning while improving the quality of the dataset.
2. Feature Recognition
AI-powered tools can identify and classify features within a point cloud, such as walls, doors, windows, or structural elements. This enables faster and more accurate drafting by automating the recognition of key components.
3. Faster Tracing and Drafting
AI can assist in tracing point cloud data to generate floor plans, elevations, and sections. Advanced algorithms can automatically align points to form clean, precise lines, drastically reducing manual drafting time.
4. Improved Accuracy
Machine learning models are trained on large datasets to recognize patterns and improve their accuracy over time. As a result, AI-generated drafts often achieve higher precision than traditional methods.
5. Real-Time Collaboration
Cloud-based AI platforms enable real-time collaboration between team members. Stakeholders can view and refine drafts simultaneously, ensuring alignment throughout the drafting process.
Applications of AI in Point Cloud to 2D Draft Conversion
The integration of AI into point cloud workflows opens up numerous possibilities for various industries. Here are some common applications:
1. As-Built Documentation
AI accelerates the creation of as-built drawings, ensuring that accurate documentation is available for renovations, restorations, or compliance purposes.
2. Large-Scale Projects
For infrastructure projects or large commercial developments, AI reduces the time required to process vast datasets, enabling faster project timelines.
3. Historical Preservation
AI facilitates the creation of accurate 2D drawings for historical sites, allowing for precise restoration efforts without damaging original structures.
4. Construction Planning
By providing quick and accurate drafts, AI supports better planning and coordination among architects, engineers, and contractors.
Key AI Technologies in Point Cloud Processing
Several AI-driven technologies are driving advancements in point cloud processing. These include:
1. Machine Learning
Machine learning models can be trained to recognize patterns in point cloud data, improving their ability to classify objects and extract relevant features.
2. Computer Vision
Computer vision algorithms analyze point cloud data to identify geometric features, enabling faster and more precise drafting.
3. Neural Networks
Deep neural networks are used to automate complex tasks, such as tracing and aligning point clouds, with high levels of accuracy.
4. Cloud Computing
Cloud-based AI platforms allow for the processing of large datasets in real time, enabling teams to work collaboratively on projects from anywhere.
Future Implications of AI in Drafting Workflows
The adoption of AI in point cloud processing is reshaping the AEC industry. As these technologies continue to evolve, we can expect:
1. Enhanced Efficiency
AI-driven workflows will significantly reduce the time and resources needed for drafting, allowing teams to focus on higher-value tasks.
2. Democratization of Tools
With AI simplifying complex tasks, smaller firms and less experienced professionals will gain access to advanced drafting capabilities.
3. Better Collaboration
AI will enable seamless collaboration across disciplines, ensuring that all stakeholders are aligned and projects are completed more efficiently.
Conclusion
Artificial intelligence is revolutionizing the way point clouds are processed into 2D drafts, offering faster, more accurate, and cost-effective solutions. By automating data cleaning, feature recognition, and drafting, AI empowers professionals to tackle complex projects with ease. As these technologies become more accessible, they will play an increasingly vital role in shaping the future of the AEC industry.